Reading with Algorithms
The US translation market is notoriously difficult to measure, but common publishing knowledge contends that only about 3% of books published in the US are translations. If you are interested in fiction only, this number falls to less than 1%.1 Between 2010 and 2021 about 8,200 translations into English were published in the US. Amazon Crossing, founded as an imprint of Amazon Publishing in 2009, published 451 of them, becoming the leading US publisher of translations into English. The second leading translation publisher during that time was Dalkey Archive, founded in 1984, which published 291.2
Amazon Crossing is such a successful publisher of translations because it has taken advantage of access to the user data of the largest bookseller in the US, Amazon.com — user data that has been collected and processed algorithmically. Although Amazon reviews, first introduced in 1997, represent qualitative data, the corresponding five-star rating system opened the door to the quantification of users' engagement with books. Quantification translates human readers's preferences into a form legible to algorithms, which facilitates an algorithm's autonomous, data-driven decision-making. Amazon's Kindle similarly allows the bookseller and publisher to gather numerical data on reading speed and perceived interest measured in the number of pages turned. Amazon has increased its data-gathering arms with its 2013 acquisition of Goodreads.com and its development of the Echo smart speaker in 2014. As of 2010, Amazon's servers housed around 82 billion data objects and responded to six million client requests per minute, and these numbers have only gone up.3
The collection and processing of this data is made possible in part by machine learning. Machine learning algorithms are programmed to achieve a particular goal, such as to get a user to buy a recommended book, or to click on a recommended book five percent of the time, or simply to show the user a recommendation that will keep them on the site longer, producing more data points. Part of the lure of the algorithms is not only that they can "think" of vast amounts of data at once (such as the entire catalogue of books available for sale in English), but also that they can conceptualize patterns in human behavior that are imperceptible to humans themselves. Because of this, it would be folly to instruct an algorithm on how to reach these goals. Instead, a machine learning algorithm uses feedback from user actions — buying or not buying, clicking or not clicking — to re-weight its inputs in an effort to produce the desired results.
The result is that algorithms are constantly being made and remade by the clicks of users, engaging in a sort of recursive co-authorship: while users read the outputs algorithms present them with, algorithms read users' reactions to those outputs — reactions and actions that become new inputs. The data that Amazon Crossing uses to make publishing decisions is algorithmically processed in the sense that it is the result of this double negotiation between users and algorithms.
While algorithms process data through a double negotiation — moving between their datasets and the inputs of users — this essay reads with algorithms by moving between the broader conditions of production of works translated by Amazon Crossing and a specific novella published in English translation by Amazon Crossing in 2015. What I hope to foreground with this movement is how interpretation — a method whose value is being contested within institutions of higher education and literary studies itself — is an attempt to make a subject of an object, turning a collection of data points describing a book-commodity legible to a search algorithm into a meaningful text that speaks to something about the humans that we are.
Although Nowhere to Be Found by Bae Suah, first published in South Korea in 1998, just a few years after the founding of Amazon, is not a direct critique of algorithmic culture, its concerns reflect questions of sociability, intersubjectivity, and identity, which publishing using algorithms also brings to light. These questions are staged primarily through the narrator's objectifying "algorithmic gaze" — a perspective that Jonathan Cohn describes as a gaze that "teach[es] us to see ourselves as if algorithmically generated and modular by design."4 When we first meet the narrator of Nowhere to Be Found, who is never named, she describes her work life as plodding on "at a measured pace," and herself as becoming objectified by her labor: "while I was busy not having any conscious thought, I became a cog."5 These observations introduce not only the narrator's life but also the style of the narration, which, at a measured pace, aims to present the reader with an objective account — from the perspective of "a cog" — of her life in 1988: her job, her family life (her father is in jail for corruption, her mother drinks, and her brother is moving to Japan because he can't find work), and her underwhelming relationship with Cheolsu.
Because algorithms deal in data, they are supposedly impartial, unbiased, and neutral, a position that gives them their cultural authority, or we might even say, offers users a new epistemology — a way of knowing unsurpassed in its objectivity. Yet recommendation algorithms also give users a sense that the output returned is the result of a democratic process. The "crowd" of data that feeds algorithms is related, in this way, to Adam Smith's invisible hand. Controlled by everyone and yet no one in particular, like a commodity price at market, algorithms' outputs give users access to the opaque workings of the hive mind — a collective intelligence inaccessible except through mass aggregation and computation. Accepting the recommendation of an algorithm as containing privileged knowledge about ourselves is akin to accepting the result of a market as reflecting a truth about human nature.
This split desire — objective access to truth and heightened knowledge of the self — describes Suah's narrator. Despite her observational narration, in many scenes, the narrator tries to discover the self that she thinks she is. At the dinner table with her family, she anticipates a future in which she is able to find that self: "I would in the end encounter that other me in the mirror."6 This "other me" — the one who is not narrating and has not become a cog — is staged here as an abrupt recognition, visible only in a mirror, that is, a surface that refracts and simulates an objective reality but is not identical with it. Like an algorithm, which, as Eli Pariser writes, is "a kind of one-way mirror, reflecting your own interest while algorithmic observers watch what you click," the narrator observes a future encounter that offers a superficial recognition of the subjective self she is looking for.7
The objectivity of an algorithm — like, ultimately, the objectivity of Suah's narrator — is illusory. Beyond the foundational problem of having no way to measure the objectivity of algorithmic outputs (although we can easily judge when they present us with biased outcomes8), it is not or at least not yet possible for algorithms to shed the impact of human intervention in their development. As developers design programs to solve problems, they make decisions (ones we would call "subjective") about, for example, "defining features, pre-classifying training data, and adjusting thresholds and parameters."9 Furthermore, the translation of human inputs into language understandable by algorithms, like all acts of translation, makes possible a gain, but also incurs some loss. Whatever output results from these data points is computed based on a radical simplification of the world, one that potentially enacts a violent rewriting of the reality that algorithms help shape.
Subjectivity is in fact written into contemporary algorithmic functioning, specifically through Bayesian statistics. Where frequentism (one hundred flips of a coin) results in an (objective) abstraction that aims to hold for a whole group or population, Bayesianism, according to Justin Joque, "allows the production of a nearly infinite field of hypotheses that can create an abstraction for each case."10 In other words, it takes advantage of the power of computation to present results that are always local, can be recalculated for each new case (or new user), and reflect a changing degree of belief over time. A probability distribution is then assigned based on the quantification of that changing belief. However, as Joque says, since there is an economic logic baked into Bayesian statistics that works to minimize loss, this quantification is calculated based on the most valuable option. Algorithms "reflect not the world as it is," Joque warns us, "but rather the world as it is profitable."11 Again, like the price of a commodity at market, an algorithmic recommendation based on Bayesian statistics is not right or wrong, but it is an objectification of social relations.12 Algorithms, then, though not objective, do objectify, and what they objectify is our relationship to ourselves and to one another.
Although search algorithms purport to give us access to a vast inventory (often called "the long tail"13) we intuitively know that searching on Amazon decreases spontaneity — the happy, accidental discovery made while browsing physical bookshelves — in book purchasing. It is harder to see, however, that decreasing spontaneity, otherness, and difference are implicit in programmed aims of algorithms. When you type into Amazon's search bar, the algorithm at play uses your search query in addition to other data points — things it knows about you (the "you" represented by your login credentials or even your IP address), as well as what other users with similar search queries have clicked on — to anticipate what you might be looking for. Using "item-to-item filtering" — data points that connect items to other items that are similar to them— search results present you with items designed to please the buyer the algorithm thinks you are based on your past purchases. Novelty — suggesting a book "out of left field" — is too great a risk for an algorithm (which, after all, has a quota of clicks to reach), leading to a sort of manufactured equilibrium. As Taina Bucher records one YouTube user saying, algorithms "can't predict for you in the future, it just assumes you are going to repeat everything you've done in the past."14
Search engines, like the one on Amazon, are incentivized to provide users with a mirror of themselves, which, ultimately, decreases diversity in bookselling, and, when that bookselling data is used to select translations, also in book production. Rather than an expanded online literary community, what we are left with is, like the mise en abyme created by looking at oneself through two mirrors at once, paradoxically intense customization and personalization on the level of the individual. The clearest way to see this is on Amazon's home page, which, since 2000 is unique to each logged-in user and presents them with items related to ones they've already purchased: the screen-as-mirror offers us a version of ourself that has been reified in data points.
Nowhere to be Found does ultimately stage for us the narrator's true encounter with her self — not through a mirror, but through a window. The scene is foreshadowed early in the novella in the future tense: "I'll cry out in the end and weep for fear of leaving this world without ever once discovering the me inside me," the narrator says, "but then I see her: another me passing by like a landscape of inanimate objects outside the window of the empty house." "Where have you been all this time?" she asks this other self.15 When the scene finally occurs in the concluding pages of the narrative, the narrator tells us that "it is my first time encountering myself."16 Unlike the view through a mirror, this encounter is not a misrecognition of the self, but a perspective on the self as it moves through the object world. That this perspective comes from inside a house, framed — like a mirror — by a window confronts us with the narrator's necessarily limited observational perspective. The "distant me," which is what the narrator calls this "precious and beautiful self," can walk freely into and out of frame, while the narrator, who exists only as an observer of the object world she is describing, remains stationary, at a distance. The important observation that the narrator makes here — and the one elided by claims to algorithmic knowledge of human behavior — is that observing the narrator's self and movement through the world is not the same as encountering her subjectivity.
User-based collaborative filtering — the "users like you also purchased. . ." recommendation engines — fulfill many of the same core functions as search engines, but they attend to these goals within a unique rhetorical stance that compels further scrutiny. When item-to-item filtering presents you with items similar to those you've already bought, it risks missing the nuances of human consumption. If you buy a toothbrush, the last thing you need is another toothbrush in a similar color and bristle strength. If you've just bought the newest edition of Alice's Adventures in Wonderland, it's unlikely that your interest will be piqued by every other edition of the book and every movie and song with the same title or publication date. A human, of course, would know this.
In contrast, user-to-user collaborative filtering, as Bezos himself translates it, "is a statistical technique that looks at your past purchase stream and finds other people whose past purchase streams are similar. Think of the people it finds as your electronic soul mates. Then we look at that aggregation and see what things your electronic soul mates have bought that you haven't. Those are the books we recommend. And it works."17 In user-based collaborative filtering, it is not objects that are being categorized and matched, but "subjects."
This distinction is particularly important since it retools the source of an algorithm's cultural authority: from mathematical objectivity to an immediate and perfect intersubjectivity. In fact, observers are quick to see this sort of recommendation engine as "facilitat[ing] interpersonal communication between customers,"18 despite the fact that no two people ever communicate in the process. A study from 2008 finds that consumers are more likely to show interest in a book labeled "customers who bought this book also bought" than one marked "recommended by the bookstore staff."19 User-based collaborative filtering appears to offer the user the best of both worlds: the authoritativeness that comes with the "objectivity" of data, and a sense also of connectedness to other human beings.
To what extent does the computer "know" you and the user it claims is like you? The answer: in an extremely limited way. Algorithms read human behavior as data points — clicks, purchases, and ratings that add up to a profile. Take for example, data collected by Melanie Walsh and Maria Antoniak, who record that as of 2020 Goodreads users were 77% Caucasian, 9% Hispanic, 7% African American, and 6% Asian.20 These demographics, however, are not entered by users; they are predicted by Quantcast based on user data. But race labels attributed by algorithms have little relationship to how we unevenly experience race in the real world. The purpose of assigning a user a race is only to be able to better market to them; the label suffices if the user makes purchase decisions that match the clicks and purchases of other users categorized under that label relatively well.21 In fact, there is what we might call a natural limit to the accuracy an algorithm can achieve in predicting a user's preferences, because user preferences themselves — like the category of race across space and time — are not stable. If you don't reliably give the same rating to the same item every time, then the algorithms are operating on faulty data.22 Although algorithms often offer results that seem uncannily attractive to ourselves as consumers, the binarism of data simply cannot account for the messy, fluid, subjective beings that we are. Again, the translation of the subject into object necessarily entails some loss.
In Nowhere to Be Found, the unknowability of the narrator's true self is repeated and heightened in the narrator's description of her lackluster love interest, Kim Cheolsu, whom she describes as highly ambivalent. Regardless of what sort of film or performance Cheolsu watches, the narrator tells us that his assessment of whether or not he likes it is always the same: "I'm not really sure."23 Cheolsu's inability to communicate his own preferences leaves the reader unable to form a strong sense of who he is and whether or not we (or our narrator) are like him. This dilemma is not resolved as the narrator describes Cheolsu's bedroom, a setting that narratives usually present as a metonymy for the characters to whom they belong. In Cheolsu's bedroom, the narrator finds "a desk, a bookcase crammed with economics textbooks, a wardrobe, a bed, a pair of dumbbells: it was the same stuff you would find in any guy's room. In the low light I perused Cheolsu's books. I didn't recognize any of the titles. He didn't have any of the usual paperbacks or light essay collections." Although just a sentence later, the narrator tells us that she "had known Cheolsu since high school," we are left wondering to what extent she really does know him.24 What does it mean to know another person and can you do so through their belongings? Cheolsu is both too easy to categorize in that he has the same stuff that any other guy might have, and too difficult to place based on the narrator's objective observations when she doesn't recognize the titles on his bookshelf. Like an algorithmic identity, Cheolsu becomes a profile, whose subjecthood is only legible insofar as our narrator can make sense of his preferences.
In the novella's central climax, the narrator attempts to visit Cheolsu, at the military training camp. After taking multiple buses to multiple locations at the camp, the narrator is told that Kim Cheolsu is not there; he's been in an accident. But there is another Kim Cheolsu who might be at the PX that she's already visited. Not knowing whether her Kim Cheolsu was the one in the accident or not, she returns to the PX to in fact find the man she had been looking for. But the confusion of the doubling persists as Cheolsu denies that there ever was a second Cheolsu at all. "Cheolsu," the narrator insists, "are there two Kim Cheolsus here?"25 This moment, which echoes the narrator's desire to encounter her real self, presents us again with the unknowability of Cheolsu. John Chenney-Lippold argues that when an algorithm hails a person — when it speaks to the "you" of the "you may also like" — it is speaking to you as a category-based profile.26 When the narrator says Cheolsu's name, she intends to signify an individual subject, but the doubling in the narration articulates the anxiety that the personal signifier — like data processed by an algorithm — unsatisfactorily translates this subject into language.
This inability to grasp the human in a constellation of data points is only the first layer of our misrecognition of the other in user-to-user collaborative filtering. A second is that these data-constellations that are presented to us as other subjects are themselves being algorithmically shaped. For example, if a user who is "similar to me" bought Through the Looking Glass after buying Alice in Wonderland, this might be because they themselves were recommended this book by an algorithm. These datafied versions of ourselves are "utterly overdetermined, made and remade every time [we] make a datafied move."27 While we may think that the choices we deliver to algorithms as we navigate Amazon's site are made from limitless possibilities, in actuality algorithms are disciplining us by giving us "a narrowly construed set [of options] that comes from successfully fitting other people." In order to operate effectively, algorithms need "stable categories of people who have learned to say certain words, click certain sequences and move in predictable ways."28 Affinities in the data don't objectively rise to the surface; they are made and manufactured by the operation of algorithms themselves.
Shortly after predicting that ultimately she would "encounter that other me in the mirror," which in many ways is what algorithms afford us, the narrator laments "the prison of a code untranslatable into the language of the other."29 The unknowability of the self through objective narration becomes the inability to articulate the self to others, particularly in the predetermined categories of language. These are the limitations that the narrator's foil, her much younger sister, aims to transcend when she says that she wants to be a lesbian when she grows up: "then I'll be in a whole new world. There's got to be something completely different out there—not just what our eyes can see."30 In fact, a whole new world — an extensive back catalogue of books impossible to access in a brick-and-mortar bookstore — is what Amazon purports to give us access to with its algorithms; the question is whether or not they do or whether, instead, they just reflect mistranslated and hyper-disciplined versions of ourselves back at us.The doubling of the narrator and of Cheolsu, which creates a crisis of describing observable selves using limited codes, is staged again on the very Amazon.com page that sells Nowhere to Be Found. Under the image of the cover, the site invites us to "Follow the Authors" by presenting us with two links. Instead of finding listed, as we might expect, the author and the translator, Sora Kim-Russell, we find the author's name listed twice with two different spellings — Bae Suah and Bae Su-ah. What would it mean to say that one of these is the page that most closely gives us a representation of the real person who is the author of this novella? Are there two (or perhaps more) Bae Suah's here?
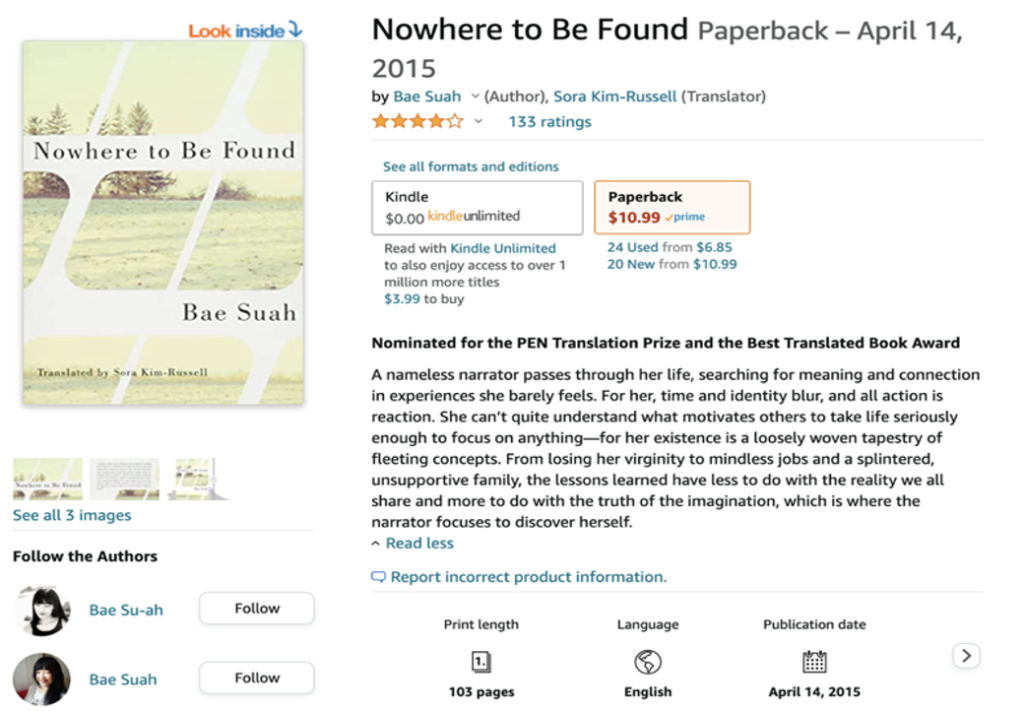
The translation of human subjects into data profiles, which are in fact shaped by algorithms themselves, reaches its apex in the misrecognition of the sum of these profiles as resembling or functioning as something that we might call a public. When Amazon recommends a book that a customer like us bought, it is, in Tarleton Gillespie's words, "invoking and claiming to know a public with which we are invited to feel an affinity."31 If it does any such thing, the public it knows is an impoverished one it has itself created, made up of subjects defined only by their purchasing histories and disciplined into categories legible to the machine. In Angie Waller's artist book Data Mining in the Amazon, she presents snapshots of what these "publics" look like through collaborative filtering recommendations. The results show that people who bought Mein Kampf often also bought the Shrek soundtrack.32 Electronic soul mates indeed. This correlation is presented as an affinity that our human cognition can't comprehend, but which is objectively true nonetheless. In reality, while user-to-user collaborative filtering asks us to "mistake the structure of somebody else's mind for our own,"33 this "user like you" is not someone we should misrecognize as a "somebody." The rhetoric of a pure, unadulterated intersubjectivity is belied by algorithms' objectification of our subjecthood. Ultimately, your electronic soul mate doesn't have a soul at all.
The specter of others — who are as illegible to the narrator as she feels to them, not to mention to herself — appear frequently in Suah's novella. The narrator pictures a "subway train at night packed with people I used to know and random people whom I will meet by chance in some distant future," who "walk by apathetically, their faces gloomy beneath the dim lights of the city hall subway station."34 The inability to "know" people in combination with their randomness renders them indistinct — their faces only dimly lit in this imagining. She even pictures her family as "just a random collection of people I knew long ago and will never happen upon again, and people I don't know yet but will meet by chance one day."35 The elements of chance and randomness as well as the passage of time coincide here to heighten the unknowability of these "others" who may be "like her" — in fact, they may be her family — but it seems there is no way to be sure.
If we see how these descriptions are presented through the narrator's objectifying, "algorithmic gaze" then these groups of strangers, although unknowable, become a static element in the text, one stranger interchangeable for another. The narrator's description of these others into obscure categories — "they were family, and they were the unfamiliar middle class, and they were malnourished soldiers. They were each other's toilets and strangers and cliffs and crows and prisons. They were never anything more than who they were"36 — is what Florian Cramer calls "the reduction of audience members to countable numbers — data sets, indices" which manifests a "self-fulfilling prophecy of stability."37 In Nowhere to Be Found, the narrator uses"third person random" to name the experience of seeing and recognizing people as a mass that appears as dimly lit objects. The phrase uncannily captures the experience of being recommended a book based on the preferences of "users like you."
Human subjectivity might qualify as the example par excellence of what Emily Apter calls the untranslatable, that which moves, "often with tension and violence — between historically and nationally circumscribed contexts to unbounded conceptual outposts; resistant yet mobile."38 And it is often precisely the untranslatable that motivates the act of translation in the first place — in this case Amazon's desire to replicate and improve on human recommendation. Yet algorithms are only useful when their processes and results exceed our comprehension — when they speak a "code untranslatable to the language of the other," i.e. us. To privilege an algorithm's understanding of us, as we do when we prefer its recommendation over another human's, or when we use its data to make publishing decisions, is to prefer an understanding that mystifies us to each other while simultaneously objectifying our social relations.
My method — moving between book and reader as objects of algorithmic processes and the novella as figuring the limits of objectifying human thought — aims to show the shortcomings of algorithms' translation from subject to object, while modeling how close reading engages an inverse process. In order to do so, I've relied on knowledge created in both humanities and STEM departments. What is at stake in the manufactured institutional conflict between these areas of study is the status and value of subjective knowledge, by which I mean both knowledge that can only be evaluated subjectively and knowledge of subjectivity. Algorithms, as I've described them here, are mechanical procedures of selection and evaluation that work to replace human evaluation with quantification of human subjectivity. Reading with and against algorithms offers an opening for how a critique of algorithms' mistranslations might be parlayed into a defense of the status of knowledge that reading literature produces. To liberate subjective evaluation from its algorithmic black box is a valuable aim for literary studies because, despite what the creators of algorithms might suggest, and as Nowhere to be Found shows us, human subjectivity cannot be objectified without loss.
***
As of November 2023, Suah's novella has 327 global ratings on Amazon and 3.6 out of 5 stars. The reviews, which represent non-quantifiable interactions with the book, often articulate confusion: "I am not sure what I just rwad [sic]" and "Unusual story. But I tried" and "Don't know why she wrote this book," a statement that indicates a thwarted, yet attempted intersubjectivity with the author. A three-star review positively recommends the novella's "Asian perspective." A five-star review provides cultural context for its readers, telling us that "the book's original title is Cheol-su, the name familiar to anyone who attended elementary school in Korea." "The name," the review continues, allowing us to perhaps recalibrate our own categorization of the character, "belongs to a young boy in the Ethics textbook used by all students. He is a model-child of moral righteousness, so immaculate in his behaviors approved by Korean social norms."
Another exuberant five-star review focuses on the high quality of the translation, but then hedges a bit: "Well, of course this is my subjective opinion."
Anna Muenchrath (@annamuenchrath) is Assistant Professor at the Florida Institute of Technology. She is the author of two books forthcoming in 2024: one on selling books with algorithms on Amazon.com (Cambridge University Press) and another on US literary institutions and the making of world literature in the twentieth and twenty-first centuries (University of Massachusetts Press).
References
- See "About Three Percent," http://www.rochester.edu/College/translation/threepercent/about/. [⤒]
- Data on Amazon Crossing and other publishers' translation is from Chad Post's Translation Database: https://www.publishersweekly.com/pw/translation/home/index.html. The analysis of this data is my own. [⤒]
- Ted Striphas, "The Abuses of Literacy: Amazon Kindle and the Right to Read," Communication and Critical Cultural Studies 7, no. 3 (January 1, 2010): 304. [⤒]
- Jonathan Cohn, The Burden of Choice: Recommendations, Subversion, and Algorithmic Culture (New Brunswick: Rutgers University Press, 2019), 158. [⤒]
- Bae Suah, Nowhere to Be Found, trans. Sora Kim-Russell (Amazon Crossing, 2015), 8. [⤒]
- Suah, 79. [⤒]
- Eli Pariser, The Filter Bubble: What the Internet Is Hiding from You (London: Penguin Books, 2012), 3. [⤒]
- See Safiya Umoja Noble, Algorithms of Oppression: How Search Engines Reinforce Racism (New York: NYU Press, 2018) for examples and critique of algorithmic bias. [⤒]
- Jenna Burrell, "How the Machine 'Thinks': Understanding Opacity in Machine Learning Algorithms," Big Data & Society 3, no. 1 (June 1, 2016): 3. [⤒]
- Justin Joque, Revolutionary Mathematics: Artificial Intelligence, Statistics and the Logic of Capitalism (London New York: Verso, 2022), 183. [⤒]
- Joque, 175. [⤒]
- Joque, 156, 175, 23. [⤒]
- Chris Anderson, The Long Tail: Why the Future of Business Is Selling Less of More (New York: Hyperion, 2008). [⤒]
- Quoted in If...Then: Algorithmic Power and Politics, Oxford Studies in Digital Politics (New York: Oxford University Press, 2018), 104. [⤒]
- Suah, 38. [⤒]
- Suah, 98. [⤒]
- "Jeff Bezos," David Sheff, https://www.davidsheff.com/jeff-bezos. [⤒]
- Nicolas Knotzer, Product Recommendations in E-Commerce Retailing Applications, Forschungsergebnisse Der Wirtschaftsuniversität Wien: 17 (Peter Lang GmbH, 2008), 2. [⤒]
- Yi-Fen Chen, "Herd Behavior in Purchasing Books Online," Computers in Human Behavior 24, no. 5 (2008): 1977-92. [⤒]
- "The Goodreads 'Classics': A Computational Study of Readers, Amazon, and Crowdsourced Amateur Criticism," Journal of Cultural Analytics 4 (2021): 255. [⤒]
- John Cheney-Lippold, We Are Data: Algorithms and the Making of Our Digital Selves (New York: NYU Press, 2017), 87. [⤒]
- Nick Seaver, "Captivating Algorithms: Recommender Systems as Traps," Journal of Material Culture 24, no. 4 (December 12, 2019): 429. [⤒]
- Suah, 25. [⤒]
- Suah, 28. [⤒]
- Suah, 73. [⤒]
- Cheney-Lippold, 91. [⤒]
- Cheney-Lippold, 31. [⤒]
- Mike Ananny, "Toward an Ethics of Algorithms: Convening, Observation, Probability, and Timeliness," Science, Technology, & Human Values 41, no. 1 (January 1, 2016): 103-4. [⤒]
- Suah, 83. [⤒]
- Suah, 82. [⤒]
- Tarleton Gillespie, The Relevance of Algorithms (The MIT Press, 2014), 188. [⤒]
- Angie Waller, Data Mining the Amazon (Self-published, 2013), unpaginated. [⤒]
- Lev Manovich, The Language of New Media, Leonardo (Cambridge, MA: MIT Press, 2001), 42; quoted in Jonathan Cohn, The Burden of Choice: Recommendations, Subversion, and Algorithmic Culture (New Brunswick: Rutgers University Press, 2019), 67. [⤒]
- Suah, 10. [⤒]
- Suah, 12. [⤒]
- Suah, 89. [⤒]
- "Crapularity Hermeneutics: Interpretation as the Blind Spot of Analytics, Artificial Intelligence, and Other Algorithmic Producers of the Postapocalyptic Present," in Pattern Discrimination, by Clemens Apprich et al. (Lüneburg, Germany: Meson Press and University of Minnesota Press, 2018), 27. [⤒]
- Emily Apter, Against World Literature: On the Politics of Untranslatability (London: Verso, 2013), 34. [⤒]